The Data Mesh and GEOSS – An unexpected precursor
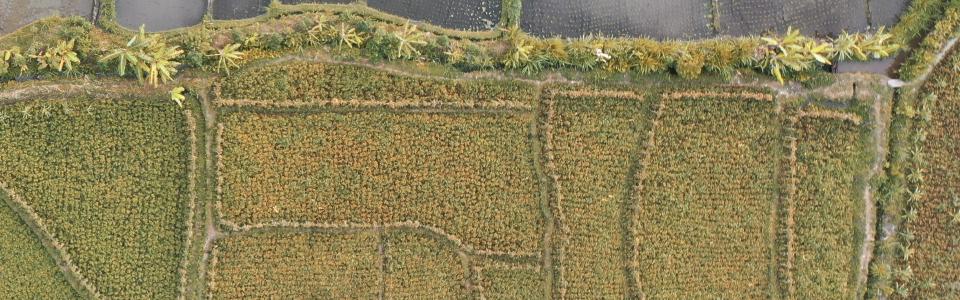
Although I’ve certainly done my share of data wrangling and analysis, for quite some time the data mesh remained yet another buzzword to me. As of late, I began to view it as what it probably is: The upcoming paradigm for data management and analytical usage of heterogeneous enterprise data.
The Data Mesh is tied to Zhamak Dehghani and her foundational work to define the data mesh as applicable principles and processes. I just didn’t know about her work as I assumed “data mesh” is yet another buzzword and went on focusing on more real issues.
But the buzzword stayed, and I decided to read up on it. But even though the clarity of concepts, diagrams and structure was great, it did not dispel an “I’ve been there before” kind of feeling.
Later, equipped with some time to reflect, it struck me that the GEOSS Platform (designed in 2005) can be considered an early implementation of the data mesh principles!
The title says “unexpected precursor” as the technology and sheer size of GEOSS are not at all mesh-like. This post is about the commonalities, and the lessons which may be derived from them.
In the following, I will assume basic knowledge of the data mesh concepts.
GEOSS, an early implementation of the data mesh
GEOSS is the “Group on Earth Observations System of Systems”. You would be forgiven if “System of Systems” sounds like microservices to you, but that analogy has been much extolled already.
GEOSS wants to bundle civil earth observation data of its members to make more effective use of it. Think satellite data, aerial photography, and derived products available internationally and the prospect of easier usage of them.
The “Group on Earth Observations” in GEOSS refers to an intergovernmental body comprised of over 100 member states and the European Commission. Thus, by definition, GEOSS is probably two or more orders of magnitude larger than most enterprise data mesh projects. So both in terms of time and space, GEOSS is a great resource to learn from for the data mesh.
Having had the opportunity to work on this amazing project for several years, I guess I’m qualified to work out the conceptual similarities between GEOSS and the data mesh. That is an interesting endeavour, as it may allow applying lessons learnt from implementing GEOSS to the data mesh. In this article, I would like to show this comparison yields useful knowledge applicable to data mesh projects.
The sheer size may serve to explain why a much earlier (2005) development project embodies the concepts of the data mesh. It seems that these concepts are practically mandatory beyond a certain scale. This could be viewed as a validation of the data mesh.
GEOSS is a data mesh for civil earth observation if you prefer. See the following table:
Data Mesh Principle | GEOSS Element |
---|---|
Domain Ownership | (SBAs, Communities & Community catalogs) |
Data as a Product | (Data Providers and Products) |
Self-serve Data platform | GEOSS Portal, DAB |
Federated computational governance | Data Management Principles, Geolabel |
I could go through the reasoning behind the table, but that is for another day. Note that map products and diverse communities have a long history in earth observation; GEOSS merely adds an integration layer. Besides these high-level conceptual similarities, many details feature a striking resemblance between GEOSS and the data mesh.
One area where this shows is the federated governance in terms of data quality. In the data mesh, governance is called upon to be “responsible for defining how to model what constitutes quality”.
This is a pretty good description of the project that introduced me to GEOSS, GeoViQua. Its objective was roughly that data quality should become metadata flowing with the data, enabling user feedback and providing incentive to improve metadata quality. Due to the nature of GEOSS, this could only work through standards such as ISO 19157. This more than matches the data meshs call to “define how to model what constitutes quality.
GEOSS might be a bit oversize, but that is not subject to this post. Size however means you should take the following consideration with a pinch of salt.
Data quality, the enfant terrible
One may define metadata on quality, quality of metadata, measures of quality, or extract quality indicators – data quality remains an elusive quantity that defies well-meant exercises to control it. Often, measures are either too simple to be effective or too complex to be applied consitently. Much effort goes to vain because of qata quality problems detected too late.
I wager that in the data mesh, data quality will not be shedding its status as the enfant terrible of data science. The data mesh assigns data quality to the data product owner, which is right, but not likely to suffice.
The root cause lies in the diversity of data and its usage patterns. Any non-trivial data quality problem will only surface at the time of (analytical) cross-domain usage. With producers and users coming from different domains, expectations towards data quality can diverge wildly. And this is where the data warehouse sneaks in, waving its common analytical data model, thinly veiled as a data quality concern against the data product.
In earth observation, this is sometimes being addressed using Data Processing Levels, but this is not a general solution.
Abstracting this a bit, quality is ultimately defined by the user of a product and data quality is no exception. Thus, user feedback mechanisms will need to be accompanied by methodical approaches toward data quality. Otherwise, one is running the risk of overloading data products with diverging user criteria, thereby re-importing data warehouse problems.
Having worked on user feedback in GEOSS, I find the data mesh a bit underdeveloped concerning data quality issues. There may be a growing need for creative approaches and solutions.
Summary
The data mesh is a modern organizational and technical approach to data management and analysis. It addresses the weak spots of its predecessors, the data warehouse and data lake approaches. It is probably a good sign that its goals are pursued with similar means on a truly global scale. Smaller projects have to be careful to select elements with actual benefit to them.
Given the comparison to GEOSS holds, we may safely assume that data quality will not be among the problems the data mesh solves or makes tractable. Shifting data quality to the producer is neither new nor sufficient. Whether this will lead to actual issues in a mesh project probably depends on the existence of beefy cross-domain use cases for the data products. Creative approaches to managing data quality issues may then be required.
Outlook
GEOSS, being an international development project, has its unique problems that make knowledge transfer to the enterprise world difficult. One cannot even hope to unify data access policy, for example. But the relative openness in which problems are discussed in this space and the similarities to the data mesh approach make GEOSS a source of inspiration beyond earth observation. Potential subjects are data citation, foreign keys, metadata quality and discoverability.
Earth observation and geographic information have always been “big data.” It is not a surprise then to see solution patterns converge as we can see in the comparison of GEOSS to the data mesh. Time will tell how “data mesh products” may support such a business transformation. When your organization is looking forward to grow beyond the data warehouse or lake, it may be worth looking at early implementations of the data mesh.